Man v. Machine: Addressing the myth of Cognitive AI
Asian Downstream Insights sits down with Sidney Lim, Managing Director of Singapore and Southeast Asia at Beyond Limits APAC, who explains how Cognitive AI differs from, well, regular AI, and addresses the “chicken and egg” situation that plagues the industry today.
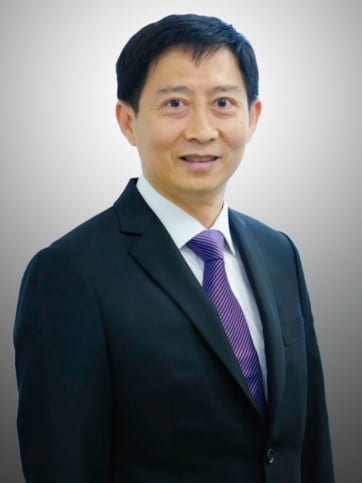
Thank you for agreeing to this interview! Please tell us a little about what you do.
I look after our Southeast Asia operations, mainly the sales and operations. Background-wise, I have been in the technology space for many years, where I started off in business intelligence data. My current role is to drive growth, build a sales team, as well as providing consultation to customers for the Southeast Asia region.
In my role, I look at delivering an AI system that has the proven ability to improve business outcomes, and advocate for a robust Data Strategy to ensure that organisations have the necessary data sources, quality, and security to successfully deploy and scale AI.
Before we get into Cognitive AI, perhaps we should discuss more conventional or less “advanced” AI. What is AI, and what role does digitalisation and AI currently play in the refining and petrochemicals industry?
When it comes to conventional AI versus Cognitive AI, the difference between the two is the data requirements. A lot of it is driven completely by business! It’s not about finding some new, interesting technology, or new kinds of experimental basis, it doesn’t matter. The bottom-line is, it’s about efficiency and productivity with the customers that we’re working with.
It’s about driving productivity and increasing profits. Businesses are driven by business objectives, as compared to a technology-driven approach, and if AI can help them drive that outcome, then you will see this piece of very interesting technology being utilised.
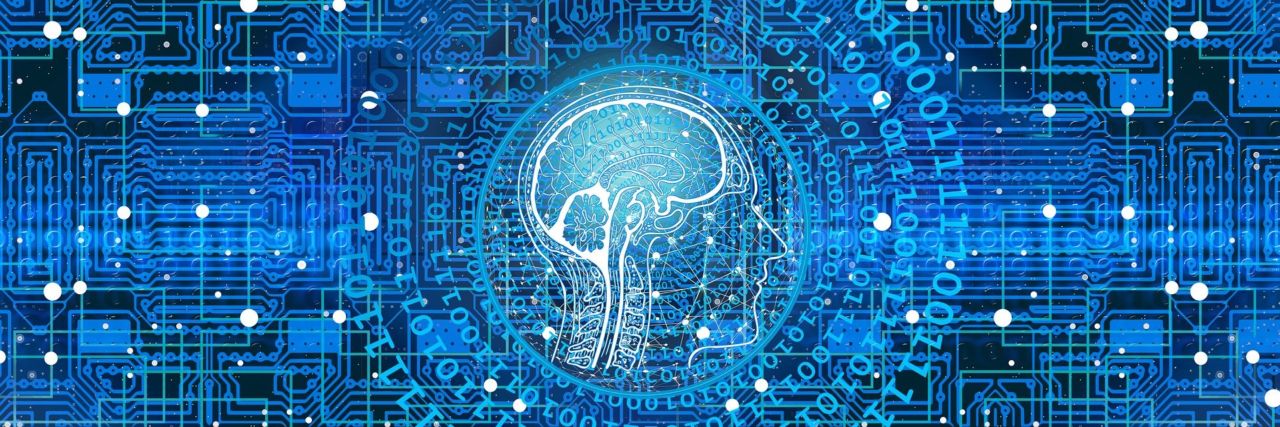
You talk about AI utilisation being a business-driven evolution instead of a technology-driven evolution; What about how this technology has evolved lately, and the rate of change that we’re experiencing today?
The past few years have been unprecedented. If I look at one of our solutions as an example, things have been tough in the last few years in terms of labour or talent. At one point, when oil prices went down, oil companies were starting to reduce their workforces and let go of people. And when the prices picked up again, companies suddenly realised they needed those extra people, and they had a hard time finding these talents.
This accessibility problem in a refinery operation is even more pronounced, considering that the talent would need experience in highly complex operations. Currently, there’s only so much that an operator can do. The experience of older operators needs to be captured somewhere or used in their absence, providing advice, and mentoring for new operators.
In this case, there is a gap in manpower where older or more experienced operators have left or been laid off, replaced by new operators who have their hands tied due to the limited experience that they can tap for different situations.
So, the refinery optimisation advisor that we have – comprising Cognitive AI – allows the system to capture human knowledge, which in this case would be the experience of the more senior operators. Taking into consideration all the dynamic factors that change refinery operations, it provides advice or recommendations for what actions to take to either correct that particular situation or optimise to maximise certain goals.
I, Robot
Right now, you’re speaking about the workflow on a more person-to-person basis, with regard to sharing data and past experiences. What about AI x Automation, and fears that the current workforce may be made redundant?
I think the myth is that one day, automation and machines and AI will replace humans – frankly, I don’t think that will ever happen. Ultimately, the refinery advisor only provides a recommendation, and cannot make the final decision. In fact, you will probably get a lot of push-back from organisations if you’re peddling a solution that will make that human decision – because of the fear of the consequences. It won’t sit well.
One good example is the topic of autonomous driving vehicles. Even with the best out there in the market, today you still hear of accidents that happen on the road. You hear about the decisions they make and consider the ethical part of the AI. If a car must go down a route, and there is a human on the left and an animal on the right, which one should the AI choose? That’s where the challenge is – having a machine make that decision.
Is this where Cognitive AI comes in?
Cognitive AI is essentially a glass box, versus conventional AI which is a black box. Conventional AI takes a whole bunch of data, churns it, and then comes up with a recommendation – we really have no clue what and how the machine comes to that recommendation. Whereas a glass box, or what we call Explainable AI, has an audit trail, and gives you the ability to figure out and understand how it came to that recommendation. With Cognitive AI, there is a human reasoning component which is set in place.
With the knowledge from experienced operators together with all these instruction manuals, equipment manuals, your standards of best practice, what we’re able to do is capture all this knowledge and put it into a knowledge base. Our system is then able to take all this data and provide recommendations for an action depending on the situation, because in an oil refinery you have a lot of variables ranging from production schedules and equipment failures to the interdependence of the various machinery, which will then impact your ability to provide output.
When you take all this into consideration, plus the experience of the operator, this feeds into the recommendation that our review system will then provide.
What are some challenges that may come in the way of implementing Cognitive AI?
The availability of data is always our first challenge. Once we’re able to overcome that, the actual adoption is a lot easier. To use one of our own examples, we’re actually in the midst of an implementation for a customer, and the first few weeks of the project were about making sure that they have the right data. Having adopted and implemented a legacy database for many years, the customer’s data sources were scattered among multiple platforms adding on a layer of complexity to the issue of available data.
In fact, much of this data was either in excel spreadsheets or from legacy databases, so it’s a matter of picking and putting that into a format that is readable and synchronized. Once that’s done, we do the crunching and configuration of the system, fine-tuning the AI algorithm, and provide the stipulated results.
I’m a little curious: You’ve previously addressed the myth of replacing redundant workers with AI. However, you’ve also mentioned Cognitive AI acts as a bit of an audit trail alongside the experience put in from human workers; where does this fit in regarding this myth?
The AI that we build is more like an advisor instead of a decision maker, and that’s because with AI – it doesn’t matter if it’s Conventional or Cognitive AI – you will never have perfect knowledge. You see a lot of applications with AI in medical science today, but I don’t think any patient (including me!) is going to rely on a decision made from a machine. We’re still more comfortable with human decisions, although with the machine, we have a reasonable recommendation made from taking in all the various data sources.
In the downstream industry, there’s a lot to consider in the refinery. Where Cognitive AI comes in is it takes the different human experiences, like the data, and combines it with people’s reasoning. Then at the end of the day, it’s a human that is making the decision after looking at the different supposed scenarios and possible outcomes.
With all this reasoning and data processing, you may still not be as experienced as the engineer with 20-30 years of experience, but even if you are someone with five years of experience, that’s still good as the AI can help you catch up.
Is there any specialized training that workers need to undertake to understand and implement Cognitive AI?
There are two paths: One is the AI technology, and the other is the subject matter. So we have a combination of the data science folks, as well as the petrochemical engineers, and they work as a team – one provides the subject matter perspective, and the other handles the technology side of things.
But one of the things that we have seen recently is a lack of talent, particularly in data science. It’s tough to take someone who’s been doing data science for the retail industry, for example, and place them into oil and gas, expecting them to be effective just because they’ve done data science. There is a certain amount of subject matter expertise required, to be able to make an impact. So that’s one of the challenges for oil and gas: It’s not sexy! People want to do medical science, they want to do pharmaceuticals, to find out the next COVID vaccine, but not many people want to work in oil and gas.
It’s a bit of a chicken and egg situation. You need subject matter experts, but to get them you need to draw in the fresh blood in the first place – which the industry is struggling with a little at the moment. That’s the kind of thing we grapple with, to help improve the industry.