Interview with Mayank Patel, Principal Applications Engineer, Siemens Process Systems Engineering, UK
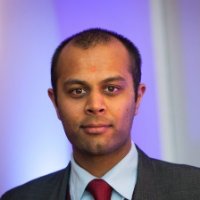
Tell us a little bit about how you got involved in the industry, and your current role at Siemens Process Systems Engineering.
Finding solutions to chemical industry problems through mathematical modelling throughout my university studies has always been a keen interest of mine. Consequently, I undertook postgraduate research activities in process systems engineering at Imperial College using tools such as gPROMS, an advanced process modelling platform.
Siemens Process Systems Engineering (SPSE), the developer of the gPROMS platform, aims to bring innovation through tools and workflows to the process industries. My role at SPSE as the head of the Application Engineering is to ensure close collaboration with our customers in the Energy and Chemicals space to maximise the use of our tools and workflows.
One of the biggest challenges the market faces is equipping the workforce to adapt to the pace of digitalisation. How do you empower your workforce to adapt to the digitalisation strategies needed to survive and thrive in the new normal?
Organisations must involve all stakeholders into the decision-making process as early as possible to support a paradigm shift in adoption of tools and workflows. Once in agreement that a shift in the way of working is necessary, they must assess site requirements in terms of functionality and users, work with operations to build, configure the desired implementation of tools.
A key part of the approach for any digital application (for example, emissions optimisation) is to go through an “operator advisory” phase, where the application provides knowledge to support informed operator decisions, before moving to closed-loop operation. This allows operators to add their own judgement to decisions and become comfortable with the system recommendations.
How can digitalisation/new technologies help organisations reach their sustainability goals?
Digitalisation in the context of process design and operation are key technologies for accelerating innovation and optimising day-to-day operation in the 21st century. Digital design using model-based technologies and methodologies makes it possible to explore the decision space rapidly and effectively, optimising equipment, process and product design, accelerating innovation and managing risk. This helps companies develop better, less polluting processes faster.
For digital operations, combining high-fidelity predictive process models that embody deep process knowledge with current and historic plant data, to create digital process twins can add significant value to plant operation on a daily basis. Virtually all digital operations are aimed at making the process more efficient, reducing raw material usage and unit energy costs, with an impact on a daily basis.
Where is artificial intelligence likely to change the downstream industry in the next five years?
Artificial intelligence (AI) and machine learning together with first-principles models can be used to structure, analyse and evaluate the huge volumes of data. This is likely to impact the chemical industry in a number of ways, such as shorten reaction times due to detection of anomalies at the earliest stage, improve yield as well as operational efficiency.
Tell us a little bit about how augmented reality and virtual reality are reducing the need to step into the plant from your perspective.
There will always be a need to step out into a plant for inspections and security. Augmented reality will support operators in the field supporting them with information from documentations systems etc., enabling them to work faster and have all relevant information at hand when he is in the plant. It will make their work in the plant more effective and might reduce their time in the plant.
Is complete virtualisation of plant and refinery operations the future?
Maybe not complete virtualisation of a plant, but virtualisation will become a bigger part of plants in the future. Having a real digital process twin that is validated and can predict the behaviour of processes can be a perfect advisor for operators in the future. Already, operations digital twins can be self-calibrating, automatically adjusting themselves to the current state of the plant before optimising operation; optimal setpoints or setpoint trajectories can be implemented in closed loop. Monitoring and soft-sensing twins provide highly detailed information on what is happening in plant equipment internally, to provide early warning. We believe that digital applications technologies combining deep process knowledge with real-time plant data will become the norm for every plant in the next 5-10 years.
How can operators get started if they want to employ the digital twin concept?
Have a clear goal on what specific task the digital process twin should solve is key. Typically, this is a business- or operations-related objective (e.g. improve overall process yield; minimise daily emissions per unit of product; etc.) rather than technology-related. One can then work backwards on what technologies and methodologies are required to address it.
Take an ethylene plant as an example, where operators may want to maximise daily yield for any given feedstock supply, taking into account the state of coking of individual furnaces. The digital twin needs to be capable of monitoring coke build-up in furnace coils, soft-sensing key variables such as ethylene and propylene yield, and optimising feed and recycle allocation between the furnaces on an hourly basis. It should also provide a “what-if” capability for analysing the effects on the product value or other KPIs of proposed operator actions, external changes, disturbances or upsets.
A digital process twin should encompass the following components:
- An underlying validated mathematical model for all scenarios that is able to predict key furnace variables such as product yield and conversion, and provides reliable real-time value for tube metal temperatures;
- Custom-configured screens that allow easy input of data and visualisation of results for operators;
- Digital platform that can fetch and post data to and from the plant DCS / historians, schedule and execute required calculations as well as monitoring the status of the digital process twin.
There is an increasing number of such twins on an increasing number of processes.
How important is culture when implementing AI into the business infrastructure? What can business leaders do to ensure that AI is accessible and understood across all parts of the business?
Clear understanding that data-driven approaches using AI or machine learning cannot displace physics and chemistry-based information available through well-established first principles approaches. Data-driven elements can certainly help to fill gaps in physics-based knowledge. However, a physics-based approach involves applying detailed, high-fidelity mathematical models of process equipment and phenomena to provide accurate predictive information for decision support.
As digital technologies are increasingly introduced into operations, in what way will they improve energy sustainability?
Digital technologies focused on managing and optimising utilities operation provides a means for refineries and chemical plants to optimise on-site production and distribution of energy to minimise cost and emissions. Application of rigorous optimisation technology to process models of the utility system, allows operators to find the economically optimal operating profile for any situation whilst taking into account demands, prices and availability, on an hourly basis or more frequently if necessary.