Clearing the Fog on Digital Transformation
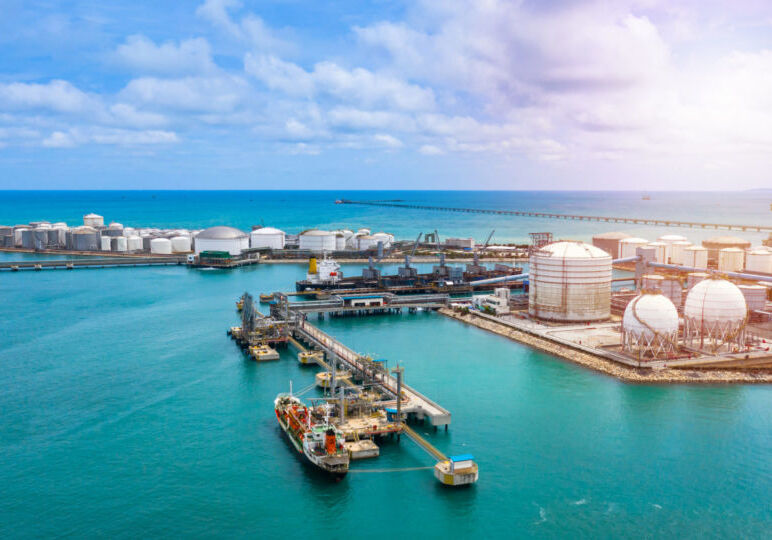
There are many manual tasks in plants which require personnel and contractors to come to a site, which prevents working from home or other remote locations. This is a limiting factor in a pandemic lockdown with social distancing. Data collection is a good example. Indeed, manual data collection has been a challenge since before the pandemic. The pandemic heightened the challenge.
The truth is that although we have lots of plant data, equipment data is missing because it is collected manually and infrequently. To achieve digital transformation, data collection must first be automated. Likewise, to employ predictive analytics, you first need the data. Therefore, advanced wireless sensors are key to digitally transforming work during the pandemic and post-pandemic. A wireless sensor network is a key technology, which typically means using WirelessHART™ inside plants.
There are two branches of AI analytics: one is machine learning (ML), which is data science and requires lots of historical data to be analyzed offline to uncover correlations and cause-and-effect relationships that are in turn used to build a model, which is then used in online mode to detect, predict, and prescribe. ML is ideal for complex problems where the correlations and cause-and-effect relations are not yet known.
The other branch of AI is readymade engineered analytics, with pre-programmed apps or templates for well understood problems, such as condition and performance monitoring of equipment like pumps and compressors where first principles and failure mode and effects analysis are already known. Engineered analytics are ideal as known solutions to known problems. Pre-engineered analytics apps improve equipment efficiency, thus reducing electricity and fuel gas consumption, losses of steam and water, and flaring and venting, thereby reducing emissions and consumption and improving sustainability.
Plants are also actively looking to meet their Corporate Social Responsibility goals. Digital solutions for occupational safety and health include emergency safety showers and eyewash monitoring, mustering headcounts, man-down rescue locating, geofencing, fatigue management, and toxic gas detection like H2S and CO. And by automating many manual tasks we keep people out of harm’s way. Other measures to make the plant a safer place to work include hydrocarbon leak/spill detection, independent tank overfill prevention, and manual valve position monitoring.
Refining and petrochemical complexes are vast and have huge numbers of assets, including hundreds of pumps and heat exchangers and thousands of steam traps and valves. Today most of these are inspected manually. Adding sensors and analytic capabilities to them can make work easier. Another challenge for refining is the great variability in feedstock, particularly high-total acid number corrosive crude and tight-oil from shale, which foul more. Adding sensors and analytic capabilities to piping and heat exchangers help refiners to decide if they are ready to take on opportunity crudes.
Energy efficiency use cases are a good way to start because the results are fast so you can show quick wins and get funding for other use cases. Use cases include steam trap failure and relief valve passing detection, equipment efficiency monitoring, and measuring energy flow on all branches throughout the plant to detect and pinpoint overconsumption.
Contextualization of data is key to filtering information to the right person and grouping related data together. For instance, data from multiple systems associated with a particular pump is grouped together so it is easy to find. Notifications are routed to those that need that information and do not disturb those that don’t. The most interesting fact is that there isn’t too much data; the real problem is that plants have the wrong data for what they want to achieve. Data scientists invariably tell you they need more data because you can’t reliably predict equipment problems using only process data. To predict equipment problems, you need equipment data. The solution is to add sensors.
Digital transformation of the plant is different from that of the office. Industry 4.0 is plant automation. It is sensors, industrial networking, chemical processes, and the process equipment domain. I&C engineers work with automation vendors to transform the plant. Start with a workshop to uncover plant challenges so you are solving real problems. There are readymade solutions for most use cases; use these off-the-shelf solutions rather than paying a consultant-integrator to reinvent the wheel.
Read more:
- HyIS-one Selects Emerson as Automation Partner for Korea’s Largest Hydrogen Refueling Station for Commercial Vehicles
- Decarbonize FCCU Catalytically
- How Natural Gas Accelerates the Path to Hydrogen
- A guide to industrial AI and digital transformation
- Case Study: Material transition to aerogel insulation leads to energy efficiency and improved thermal management