How next-gen hybrid digital twinning technology transforms refinery performance
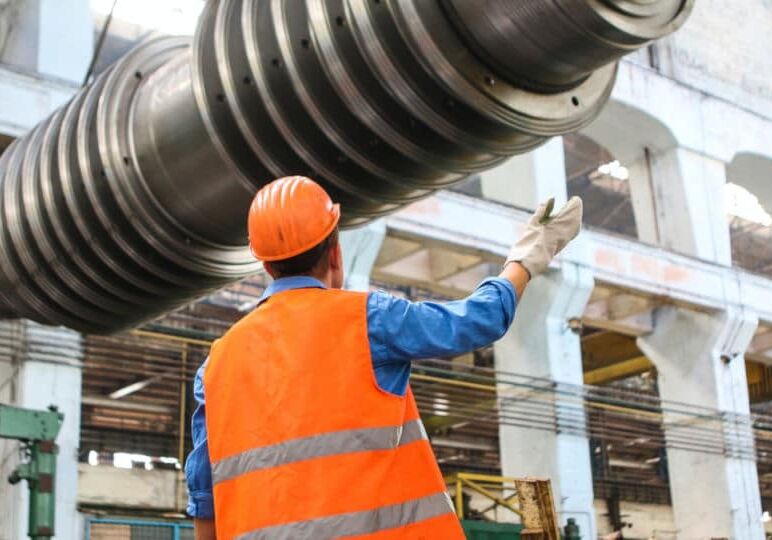
By Jari Marci & Thomas Diehl
Digital twin technology and machine learning are buzzwords – but what do they mean for refineries? Digital twins are a key component of the digitalisation journey, incorporating AI and Machine Learning, but many of us fail to fully appreciate the impact they will have in driving decision-making and maximising profit at the operating level – even for well-established process technologies.
The benefit to clients? This new hybrid approach offers the best of both worlds – resulting in streamlined operations, measurable performances, increased efficiencies and reduced safety incidents. In a post-COVID world, refineries and petrochemical operators can reduce headcount, keep workers COVID-safe, and rely on virtual expertise as never before.
Refineries and petrochemical sites have always operated according to “first principles”. Those scientific tenets dictate when you run X through Y processes. They are robust and predictive; yet in an increasingly complex market, these alone may not be enough for optimal performance.
There is even more added value in the new generation of so-called Hybrid models. They combine the robustness and predicting power of first-principle engineering models with speed and adaptivity of data models and AI-based machine learning. The lessons learned of “pure” data models without expert knowledge and engineering experience is overcome by the intelligent application and implementation of well-established balancing, process and Real-time CFD models onto a data platform.
Case Studies
Petrogenium has been called in to support refiners in navigating their digital transformations. In a recent case with a European refiner, the prediction of the stability parameter or P-value was found to be of good quality despite considerable dead time related to extensive interim tankage. Utilising such a digital twin model as part of an online Real-Time Optimisation (RTO) can easily generate margin improvement in the order of 1.5 MMUSD/annum for a typical 2400 t/d unit (or 0.30 USD/bbl). Application of this technology opens doors, which previously had been closed. For the VBU these would be the prediction of the unit’s internal fouling behaviour (furnace skin-temperature increase as a function of non-operating and feedstock properties) as well as upset detection – aspects which to date rely on human supervision to perform optimally.
The VBU Case Study
The first example is the digital twin model developed for a Visbreaker Unit (VBU). Visbreaking technology is a widespread and old petroleum processing technology mainly used for the production of residual marine fuel oil.
Visbreaking is a widespread, tried and tested processing technology, mainly used for the production of residual marine fuel oil. Yet with a digital application, its performance can be greatly enhanced. The Petrogenium digital twin capabilities were benchmarked against real operations, with surprising results – a deviation of less than 0.2% comparing to actual unit performance.
A VBU employs a mild form of thermal conversion in order to generate gasoil components, which reduce the viscosity of the residue processed. To generate the digital twin model, process data for a typical 2400 t/d unit covering the operation of almost one year in 1-hour steps was used. In total, 130 process measurements were utilised.
In addition to physical process data, 11 different laboratory analytics as well as the feedstocks’ compositional breakdown (crude oil grades) were fed into a machine learning platform. After data analytics and parameter clustering, the digital twin model was generated exclusively on basis of the process data provided. Its capabilities for predicting process performance were benchmarked against real life operating performance, with surprising results.
The key performance indicators (KPI) predicted the unit’s conversion to light products and the P-value stability parameter for cracked residue. The prediction of the unit’s gasoil minus conversion had a deviation of less than 0.2% comparing to actual unit performance. This is an impressive feat, which would have been a challenge for many industry process simulators. These previously needed to be fed this explicit information to produce meaningful results.
Process optimisation and optimised maintenance and inspection based on these data models has already been done successfully in off-line projects for these processes in the past as well as currently on-going pilot projects with Real-Time Optimisation (RTO).
Utilising such a digital twin model as part of an online Real-Time Optimisation (RTO) can easily generate margin improvement in the order of 1.5 MMUSD/annum for a typical 2400 t/d unit (or the equivalent of US 30 cts/bbl). It offers deep process insight, with the ability to be everywhere at all times. Real-Time process analytics can identify both short-term influences and long-term drifts. By combining fast machine learning with physical engineering balance models, KPIs can be accurately calibrated and predicted.
Application of this technology opens doors to greater efficiencies and cost savings which previously relied on human supervision, providing better understanding, “what if” analyses, enhancing operational capability, and encouraging more integration. The digital asset benefits include an increase in fuel yields, consistent product quality and stability, energy efficiency and life cycle rentability. Data analytics can analyse over 140+ signals, 10+ process units and five KPIs – all at once. These can help create conversion yield KPIs, including product rate, light oil product quality, p-Value and process turnover.
The CCR Reformer Case Study
The second example is found in the petrochemical domain. For downstream CCR reformers as well as in pygas processing in ethylene plants, Extractive Distillation was implemented for the recovery of BTX Aromatics – mainly pure benzene, but also TDI grade toluene.
This process allows the separations of aromatics at high purity and the non-aromatic components in the respective feedstocks. As they have very close boiling points and azeotropes are formed, the use of a polar extractive solvent as an intermediary was necessary. All this is embedded in a closed loop distillation process showing strong non-linear behaviour and relatively long reaction times of process adjustments (lag time). In a use case for a 350.00TPA benzene unit, the recovery of benzene was increased such that an annual additional benzene production of 500,000 Euros could be attained.
Modelling the complex thermodynamic interactions of this system is highly complex and reserved to those in possession of specialised, normally proprietary thermodynamic data. Using a data model incorporating comprehensive statistics and self-learning artificial neuronal networks, this process can now be modelled and predicted using the factories own process data with an accuracy superior to even the most specialised first principles modelling tools. With this predictive capability, this process can be optimised to a performance level above and beyond first quartile performance. In the extractive distillation area, there are multiple successful applications.
Process optimisation and optimised maintenance and inspection based on these data models have already been done successfully in off-line projects for the above processes, as well as in on-going pilot projects with Real-Time Optimisation (RTO).
The Hybrid Revolution
Enter hybrid models! With AI innovations and data scraping, this next-gen digitalisation is opening opportunities for smaller and medium-sized refineries around the world who often lack internal technology networks. AI-based ML is fast, adaptable and proactive. The predictive power of first-gen engineering models is coupled with real-time operational data from ‘Digital Twins‘ and self-learning. A fully machine learning-based model is realistic and feasible. However, in some cases – such as where CFD is involved – only a hybrid model can be a catch-all.
Simulation technologies are feasible for multiple applications, from process design, CFD services and computer-aided engineering, to virtual reality, big data analyses and RealTime process optimisation. This has become a part of IoT & Industry 4.0.
Remote Operating Centres
For larger assets consisting of several locations, establishing a Remote Operating Centre (ROC) is the vision of the future, where all assets are represented and commonly optimised. Digital Twin models for processes and assets, a fully digital technical documentation and RTO routines are the minimum pre-requisites to make this vision come true. Further Machine Learning (ML) based on AI cloud operated self-improving models realised in the form of Operator Assistance Systems are the final steps to ‘close the loop‘ for fully automated operation.
By applying digital twin technology and machine learning, industry players can embrace the challenge of increasing industrial complexity and pace of the energy transition and turn obstacles into opportunities.